To Share Or Not To Share: How AIS Engage’s ‘No Secrets’ Policy Shocked the AI Industry
- Edan Harr
- Feb 7
- 11 min read
Updated: Feb 11
This AI Company's Radical Transparency Approach Has Industry Veterans Sweating: here's how AIS Engage’s ‘No Secrets’ policy shocked the AI Industry from New York through Silicon Valley.
The AI industry has long operated behind closed doors, with companies zealously guarding their algorithms and training data as precious trade secrets. Now, one rising player has broken ranks in spectacular fashion by making their entire AI development process public. In today's increasingly scrutinized AI landscape, transparency has become the elephant in the room. Tech giants face mounting pressure over their "black box" approaches, while regulators and the public demand greater accountability. This unprecedented move challenges the very foundation of how AI companies operate. After all, the standard playbook calls for strict secrecy and carefully controlled information release. But this company's "glass box" strategy is proving surprisingly effective- while established players maintain their fortress mentality, this upstart's radical transparency has led to a 200% increase in enterprise adoption compared to industry averages, with their open-source approach attracting both customers and talent.
Pioneered by a collective of AI ethicists and former Big Tech analysts, this transparency initiative represents a dramatic shift in how artificial intelligence development could be approached. At its foundation, the policy requires complete disclosure of training methodologies, data sources, and model architectures to the clients they work with. Through client specific documentation, peer review processes, and real-time monitoring dashboards, it creates unprecedented visibility into AI development. Put simply, they've torn down the walls between AI creators and users, establishing a new paradigm of collaborative development and shared accountability. The impact? A revolutionary approach that's not just changing how AI is built, but fundamentally reshaping industry expectations around transparency, trust, and corporate responsibility.

Opening Up The “Black Box”: The Tipping Point That Led To Transparency
When 78% of enterprise AI implementations stall due to vendor lock-in and lack of access, the disconnect between service providers and clients becomes painfully clear. Artificial Intelligence Solution’s transparency initiative was born from firsthand experience with these frustrations. Unlike traditional AI companies that treat their systems like fortified vaults, this approach emerged from a pivotal moment in manufacturing analytics that exposed the deep-rooted problems with secretive business practices. Built on lessons learned from a Fortune 500 manufacturing company's struggles with opaque third-party vendors, the initiative represents a complete reversal of industry norms.
Picture this: A leading manufacturing company attempts to transition between reporting systems, only to find themselves handcuffed by their vendor's secrecy policies. Their in-house analyst, who would later become a founding member of Artificial Intelligence Solutions, encountered a frustrating paradox- hired to optimize systems she wasn't allowed to access. The vendor's paranoid protection of their "secret sauce" forced the manufacturing company's IT team to operate in the dark, leading to duplicate work, delayed implementations, and compromised solutions. When a company needed custom dashboards for specific departments, they faced an absurd choice: wait months for the vendor's overworked IT team or build temporary solutions that would need complete reconstruction later. "We were essentially building everything twice," recalls the founding team member, "once in our systems and once in theirs- all because they wouldn't let us peek behind the curtain."
When she founded AIS, she was determined to avoid those same roadblocks, without compromising her business. The solution came together during a landmark AI implementation project for a major financial services provider. The client needed an AI system that could engage with high-net-worth investors while maintaining complete transparency about how their sensitive data was being processed. Traditional vendors proposed their usual black-box solutions, complete with NDAs and limited access protocols. But after the manufacturing analytics debacle, the founder recognized a familiar patter- the same walls of secrecy that had hampered previous projects were now threatening to compromise a solution that desperately needed trust and verification at its core.
The initial client meetings revealed sobering statistics: 92% of their investors expressed serious concerns about AI systems handling their financial data without transparency, while 76% of their compliance team feared hidden biases in black-box AI solutions. The project posed a fundamental question: How could they build an AI system that both protected sensitive financial information and provided complete transparency about its operations? The traditional industry approach of "trust us, it works" wasn't just inadequate - it was potentially dangerous. This challenge would become the catalyst for a radical rethinking of how AI companies approach transparency, leading to a solution that would eventually shock the entire industry.
Verifying the Source: Inside The Mind of an AI
The first major roadblock hit during the initial testing phase of our AI system. The wealth management division was testing a conversation flow where a high-net-worth client asked about adjusting their retirement strategy given recent market volatility. The AI generated a sophisticated response about portfolio rebalancing, complete with specific asset allocation suggestions. The compliance team's reaction wasn't what anyone expected. Instead of evaluating the quality of the advice, their lead compliance officer asked something that would reshape the entire project: "Show me exactly how the AI arrived at these percentages, and which internal investment policies it referenced to make these recommendations."
This seemingly simple request exposed a critical gap. The compliance team needed more than just the conversation transcript- they needed to trace the AI's entire decision path. Which knowledge base documents did it reference? How did it interpret the client's risk tolerance from their profile? What specific sections of their investment guidelines influenced the recommended allocation splits? When the compliance team tried to verify these elements, they discovered they couldn't connect the dots between the client's initial query, the internal documentation the AI had accessed, and its final recommendations. They were forced to flag the interaction for manual review, creating exactly the kind of service bottleneck the AI was supposed to eliminate. This moment crystallized the core challenge: building an AI that could not only provide sound financial advice but also explain its reasoning process and cite its sources in real-time.
Sand Under Pressure: Building The Glass Box Solution
Our breakthrough came from fundamentally rethinking how AI should interact with its knowledge base, which became a crucial part of how AIS Engage’s ‘no secrets’ policy became something that shocked the AI Industry. Instead of treating the AI as a black box that ingests information and produces answers, we developed what we call a "glass box" approach- making every step of the AI's decision-making process visible and traceable.
Think of it like watching a master chess player explain their strategy. Rather than just seeing the final move, you see their analysis of the board, the potential moves they considered, and why they chose their specific approach. We built our AI to function the same way, creating a visible chain of reasoning that shows exactly how it moves from question to answer.
The technical implementation required careful orchestration of several components. We developed a custom prompt engineering framework called the Citation and Logical Conclusion Feature that instructs the AI to structure its responses in discrete, traceable steps. Each response is generated through a series of sequential prompts:
1. Initial query analysis prompt that forces the AI to explicitly state how it interpreted the user's question
2. Document retrieval prompt that requires listing all sources it plans to reference
3. Citation extraction prompt that pulls relevant passages and assigns unique identifiers
4. Synthesis prompt that shows how these elements combine into the final recommendation
We implemented this through a chain of specialized conversation steps, each with its own carefully crafted system message that enforces our transparency requirements. The AI can't proceed to its next logical step without first documenting its current reasoning and sources. This creates a natural checkpoint system where each piece of the response is validated before moving forward.
When the AI suggests a 60/40 portfolio split, for instance, compliance officers can now trace exactly which investment guidelines led to that recommendation and how the AI interpreted them. Each step is linked to specific citations from the knowledge base, complete with timestamp and document location. The key innovation wasn't just in tracking sources- it was in making the AI's reasoning process an integral part of its response architecture. Every conversation flow is now built with transparency checkpoints, where the AI must show its work before proceeding to its next logical step.

Hidden Behind Closed Doors: The Document Traceability Challenge
But we weren’t out of the woods yet. With our Citation and Logical Conclusion feature up and running, the second critical issue surfaced during what should have been a seamless live demo. We demonstrated a client relationship manager asked the AI about tax-loss harvesting strategies for a portfolio heavy in municipal bonds. The AI generated a detailed response, complete with our newly implemented citation system showing exactly which documents it had referenced. Each citation included the specific section, paragraph, and reasoning chain- a process that initially impressed the compliance team. However, this same transparency feature revealed a critical flaw: the AI had based its core recommendations on "Municipal Bond Investment Guidelines_2022.pdf" instead of "Municipal Bond Investment Guidelines_2024.pdf."
The discovery sparked a complex debate during our project review. The compliance officer example demonstrated how our citation system had worked perfectly- it showed exactly how the AI had constructed its argument, pulling specific passages about tax-loss harvesting thresholds and linking them logically to its final recommendations. The problem wasn't with the reasoning; it was with the source material. Creating all new accurate documentation would be far too time consuming, the document naming conventions needed to be kept in a standard format for reporting purposes, and we couldn't simply delete the older documents- they contained crucial historical context about past policy decisions and some unique explanatory sections that hadn't been carried forward into newer versions. The firm needed these for audit trails and institutional knowledge preservation.
This revelation was simultaneously concerning and validating. Our transparency features had caught something that would have been invisible in a traditional black-box AI system. Without our citation and logical chain tracking, the AI could have been mixing outdated and current policies for months without anyone noticing. The only reason we caught it was because we could see exactly which document versions the AI was using to construct its arguments.
Accuracy Through Time: Finding the Fix to Document Versioning
The power of our glass box approach became even more apparent as we tackled the historical documentation challenge. Rather than creating an entirely new system, we leveraged our existing transparency framework by enhancing our conversation flow design.
We extended our AI's decision-making framework by creating sequential conversation steps that enforce version awareness. Before providing any recommendations, the AI moves through a series of structured conversational checkpoints. First, it examines stored metadata about each document, which we maintain in our knowledge base as key-value pairs. This allows the AI to understand where each document sits in the chronological hierarchy. It then uses custom variables to compare document dates within the same category, identifying any potential version conflicts or overlaps. As part of its transparent reasoning chain, it must explicitly state why it selected specific document versions, particularly when choosing between different temporal versions of the same policy or guideline.
The technical implementation built naturally upon our existing conversation design. We created two primary conversation paths that work in harmony with our transparency framework. The first path focuses on document analysis, where we use conditional logic to verify version status and relevant metadata before proceeding with any information extraction. The second path handles version selection, where custom conversation flows ensure the AI validates its source selection and provides clear justification for its choices.
This approach maintained our commitment to transparency while solving the versioning problem. When the AI now references older documentation, it must explicitly justify why it's using that version instead of the current one, storing these justifications in variables that become part of its visible reasoning chain.
The solution proved elegant because it built upon our existing glass box architecture- we weren't adding complexity, just extending our transparency requirements to include temporal awareness. Every document reference now comes with both its reasoning chain and its temporal context, allowing compliance officers to immediately understand not just what information the AI used, but why it chose specific document versions.
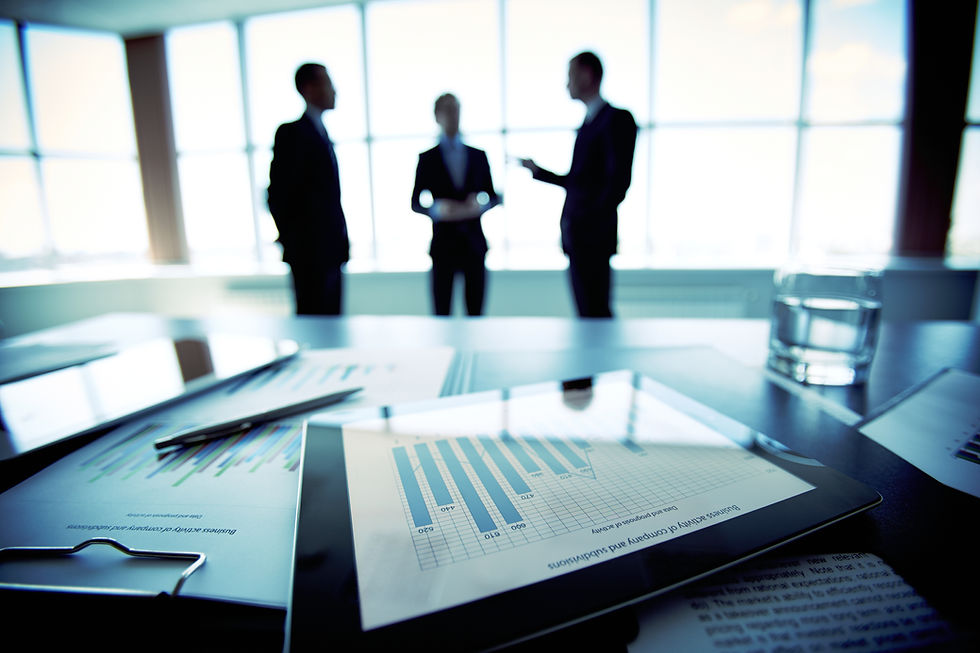
Lasting Implications: The Power of Transparent AI in Practice
What began as a solution to a specific compliance challenge quickly revealed broader implications across multiple industries. By designing sequential conversation flows and maintaining contextual awareness through each interaction, we created a system that proved remarkably adaptable to any sector dealing with complex documentation and regulatory requirements.
During our first month of deployment, we discovered our system was catching subtle policy conflicts that had gone unnoticed for years. In one instance, the AI flagged an apparent contradiction between current investment guidelines and their previous versions, leading to the discovery of an unintended policy drift that had occurred over several document updates. The transparent approach didn't just show the conflict- it demonstrated exactly how the policies had evolved over time, providing crucial context for policy refinement through carefully structured conversation paths and stored metadata.
This level of transparency began attracting attention from other organizations, particularly those struggling with their "black box" AI solutions. While competitors could provide AI-generated answers, they couldn't show how those answers were derived or which version of which documents influenced the response. In regulatory audits, this difference became stark- our system could demonstrate not just compliance, but the exact reasoning path that led to each decision through its built-in conversation tracking.
The system's impact spread across various sectors. AIS has used it for healthcare organizations to manage evolving clinical guidelines while maintaining access to previous protocols. They’ve implemented it for legal teams for case law references, ensuring associates could trace exactly which precedents influenced each analysis. We’ve adapted it for manufacturing and pharmaceutical companies for quality control and regulatory compliance, using the transparent reasoning chains to strengthen their audit processes.
An unexpected benefit emerged in document management and optimization. Teams began using the system's transparent reasoning chains to identify redundant or missing information across document versions. When the conversational flow required references to both old and new documents to answer a single question, it often revealed content that should have been carried forward but wasn't. This led to more coherent documentation across the board, as teams could see exactly where historical context was being lost or unnecessarily duplicated.
The system became a powerful tool for employee training and knowledge transfer. New team members could now see exactly how the AI approached complex queries, using the visible decision paths as learning tools. The system became a de facto institutional knowledge repository, showing not just what the current policies were, but how they had evolved and why certain decisions were made through its structured conversation flows.
Perhaps most importantly, this transparency created trust. Stakeholders appreciated being able to see exactly how and why specific recommendations were made. The ability to trace every decision back to its source documents, complete with version control and reasoning chains, provided a level of accountability that became a significant competitive advantage. When management considered policy changes, they could use the AI to simulate how new guidelines would interact with existing ones, seeing exactly where conflicts or ambiguities might arise. This predictive capability transformed policy development from a reactive to a proactive process.
A New Wave: Pioneering the Standard for AI Transparency
As we look to the future, the implications of this transparent approach extend far beyond its initial scope. What we've created isn't just a solution to document versioning- it's a potential framework for building trust in AI-driven decision making that could reshape how organizations approach artificial intelligence, large language model development, and more.
The key lesson learned is that our Citation and Logical Conclusion feature isn't just a feature- it's a fundamental requirement for AI adoption in regulated environments. By designing systems that can explain their reasoning, maintain historical context, and show their work, we've demonstrated that AI can be both powerful and accountable. This balance is crucial as organizations increasingly rely on AI for complex decision-making processes.
The ripple effects of this approach are already visible. Organizations that initially implemented the system for compliance purposes are discovering its value in policy development, training, and risk management. The ability to trace decision paths and understand document evolution has transformed how teams approach documentation and knowledge management. Looking ahead, we see several exciting possibilities:
1. Enhanced Decision Support: As organizations build upon this framework, they can create increasingly sophisticated decision support systems that maintain complete transparency while handling more complex scenarios.
2. Proactive Compliance: Rather than reacting to regulatory changes, organizations can use this approach to simulate the impact of new policies and identify potential issues before they arise.
3. Knowledge Preservation: The system's ability to maintain and explain historical context offers a new way to preserve institutional knowledge, making it easier to train new employees and maintain consistency across time.
The success of this transparent approach challenges the notion that AI systems must be mysterious "black boxes." We've shown that it's possible to create AI solutions that are both powerful and explainable, maintaining a clear chain of reasoning that builds trust with users and regulators alike. As AI continues to evolve, the principles demonstrated here- transparency, accountability, and clear reasoning- will become increasingly important. Organizations that embrace these principles now will be better positioned to adapt to future regulatory requirements and maintain stakeholder trust. By prioritizing transparency and explainability from the start, we've created something that not only solves today's challenges but provides a foundation for addressing tomorrow's needs.
Σχόλια