Building "ROME": How One AI Research Project Could Point to an Industry Wide Revolution of Technological Advancement
- Edan Harr
- Feb 8
- 7 min read
Updated: Feb 13
It may not have been built in a day, but "ROME" changed the future of enterprise AI development overnight. With its foundation in mathematics and lightweight architecture, this enhanced intent recognition system gives more computationally complex AI models a run for their money.
In an industry where the standard has been to increase computational power and complexity to achieve better results, the Recursive Optimization for Model Enhancement (ROME) framework introduced by Analytic Intelligence Solutions (AIS) has turned the tables. ROME's groundbreaking approach challenges the common industry belief that throwing more computing power at AI automatically makes it more intelligent by demonstrating that high-accuracy intent recognition can be achieved through mathematical optimization instead of brute computational force.
The breakthrough lies in three key innovations: a function that preserves the most relevant context to maintain temporal relevance, a function that adapts system thresholds to improve accuracy as intents are routed successfully, and a keyword scoring system that utilizes select metrics to determine word relevance. In practical testing across up to 108,900 daily interactions, ROME demonstrated 76ms response times while requiring only 512MB of RAM- a fraction of the resources needed by conventional systems. To put this in perspective, many current AI solutions require 4-16GB of RAM to handle basic conversations.
What makes this development particularly significant is its practical impact: ROME can effectively handle 24.63% of traditional LLM operations while using less than 10% of the typical computational resources. When researchers at AIS introduced the ROME framework, they exposed a blind spot so fundamental that major AI companies are now reassessing their entire approach. We interviewed the lead researcher for the ROME framework, Edan Harr, to get her take on the development process and its future applications to AI. This inspiring story of ROME's practical impact forces us to ask: what other revolutionary innovations might we be missing by following the conventional approach?
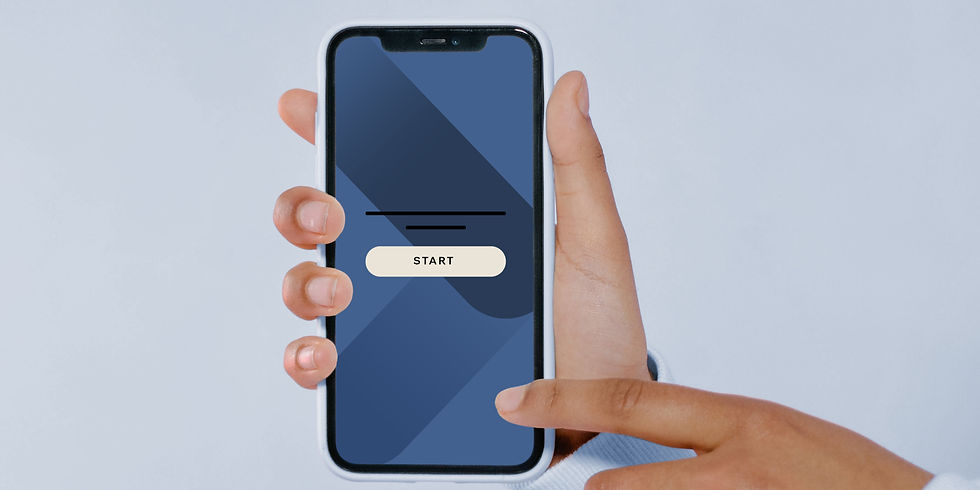
R for Recursive: The Foundation of Natural Dialogue
In computing, recursive refers to a method where the solution to a problem depends on solutions to smaller instances of the same problem. In the ROME framework, this principle is used to specialize responses based on each user interaction according to the conversational AI's understanding. ROME reassesses and pivots its approach in real-time as the user zeroes in on the core issue that needs to be solved. Traditional AI systems rely on static models that require periodic retraining to improve response relevance, but ROME avoids this by adjusting its algorithms within the active conversation.
"The real revolution isn't in the complexity, but in the simplicity. That was our whole goal when we set out to create ROME," explains Harr. "We noticed that throwing more and more pretraining at AI models and trying to create larger context windows and more advanced computing capabilities was having the opposite effect of what businesses wanted, ultimately resulting in lower success rates over time. With ROME, I think we've successfully proven that effective AI doesn't always require massive computing power or enormous datasets."
This approach challenges the industry's "bigger is better" mentality, which requires extensive computational resources and specialized expertise, leading to implementation costs exceeding $144,000 for a basic conversational chat system. ROME's framework dramatically reduced these costs by 96.67% in testing scenarios, bringing the implementation budget down to around $4,800 and achieving superior results in pattern or intent recognition tasks. This significant cost reduction without a loss of capability is direct proof of the financial benefits ROME can provide, which is exciting for the future of affordability for advanced AI solutions.
"When I show these numbers to other developers, their first reaction is usually disbelief," Edan Harr notes with a slight smile, referring to the charts in the recently published open-source ROME research paper, which is available on the AIS website to remain easily accessible to the everyday developer. "Even I go over it again sometimes to make sure its correct. But we covered all our bases. And then, they see our testing methodology, the mathematical proofs, and the real-world implementation data. They understand we're not just making incremental improvements. We're fundamentally rethinking how these systems should work."
O for Optimization: The Foundation of Natural Dialogue
Optimization in computing means finding an optimal solution regarding efficiency, performance, or resource usage. For ROME, optimization is not just about speed or resource conservation; it's about making every interaction count toward better understanding and response. Initial deployment focused on public-facing implementations across multiple websites, generating extensive user interaction data.
"People always ask about the technical specifications first," Harr notes. "But what they're really asking is: how did you make it so efficient?" The framework parameters were calibrated for optimal performance across deployment scenarios, using dynamic context preservation and adaptive thresholds that adjust based on interaction outcomes. For less technical strategic decision-makers, AIS released a one-page executive summary detailing the answer to that question.
To put it simply, ROME leverages optimization through several key strategies. The system learns from its successes and failures without manual recalibration, ensuring continuous improvement and stability. Testing showed a remarkable 99.5% uptime throughout the initial deployment period. The testing methodology included technology executives from Fortune 500 companies, enterprise AI specialists, engineers, small business owners, and experienced contract workers.
"The real breakthrough came with the pattern scoring system," Harr explains. "We wanted it to be expansive for developers to grow from there. Little tweaks would improve it for some use cases, but different issues that made it less relevant to others would pop up. We figured we'd leave the fine-tuning to the developers and try to make it as cover as many applications as possible across the board. I like what we came up with in the end."

M for Model: The Foundation of Natural Dialogue
In AI, a model refers to the conceptual structure used to simulate real-world phenomena. ROME redefines this by introducing a model in which the architecture itself evolves recursively with each interaction rather than being a static entity that needs massive data to adapt.
The environmental impact of this breakthrough extends to energy consumption. Current data centers require massive amounts of water for cooling, with some large-scale AI training operations consuming over 500,000 gallons of water. Based on ROME's computational efficiency, widespread adoption could significantly reduce this consumption. Initial projections suggest that if just 25% of current AI operations switched to ROME-based architectures, the water savings could equal the annual consumption of a small city.
"We're clear about what ROME can and can't do," says Harr. "It's not about replacing LLMs; it's about using the right tool for each job. Even if you can replace 25% of your current system with something more lightweight and cost-effective, that's more than 0%." This reassurance about ROME's adaptability instills a sense of confidence in the audience about its ability to handle diverse AI tasks.
E for Enhancement: The Art of Guided Conversation
Enhancement in ROME isn't just about making things more effective- it's about making them more intelligent. The system adapts based on its success rate, much like how humans learn from experience. If targeted approaches work well, it reinforces them. If others don't perform as expected, it adjusts accordingly. This creates a natural learning curve that doesn't require massive computational resources or complex algorithms.
"When developers see our approach, they often expect complexity," says Harr. "But we've intentionally kept everything straightforward without losing any accuracy. We actually get higher accuracy rates most of the time." Think of it like a skilled conversationalist who remembers recent discussions clearly, while older conversations naturally fade but never disappear entirely.
"What makes ROME different is that it doesn't try to know everything at once," explains Harr. "Instead, it focuses on understanding the immediate conversation and learning from each interaction. It's much closer to what we'd expect in a human-to-human conversation, where it treats every piece of what a user says as context and fills in responses from there."

The Rise of ROME: A New Standard in Conversational AI
ROME represents more than technological advancement; it marks a shift in how we approach artificial intelligence development. Traditional AI research and deployment have belonged to companies with enough money for resource-intensive solutions, creating barriers for smaller organizations and limited innovation scopes. ROME creates an opening for developers, businesses of all sizes and stages, and everyday users to access customizable, advanced AI systems with minimal setup.
"What excites me most isn't just the technology. It's seeing small teams implement ROME and achieve results that previously required massive resources. We're democratizing AI in a way that actually works. We're at a turning point," Harr concludes. "The future of AI doesn't have to be about bigger models and more resources. ROME shows that we can build better systems by being smarter about how we use what we have. That's the real revolution."
Early adoption statistics show promising trends. Small to medium-sized businesses report implementation times averaging 3-4 weeks, with teams as small as two developers successfully deploying ROME-based systems. The framework's efficiency has also caught the attention of larger organizations, with several Fortune 500 companies already beginning pilot programs in and out of their current AI systems. The open-source nature of ROME has built a growing community of developers who are building upon and expanding its capabilities. Over 300 independent developers and tech executives contributed improvements and adaptations within the first six months of testing, providing valuable data relevant to fine-tuning ROME for specialized industries.
When asked about the next steps, the AIS development team believes that ROME's impact extends beyond its technical success. Its lightweight approach to building AI systems could reshape current industry standards, making sustainable ML systems the standard rather than the exception. As concerns mount over AI's massive resource consumption, ROME's efficient design offers a practical path forward for companies interested in reducing their environmental footprint without losing intent recognition accuracy. As concerns about AI's resource consumption grow, ROME's lightweight footprint offers a viable path forward.
Comentarios