Bridging the generation gap between traditional metrics and modern AI capabilities reveals how AIS Engage built the next generation of business analytics, transforming enterprise intelligence from a historical record into a predictive powerhouse.
"Robert Anderson." "Bob A." "Anderson the Manderson." Three names, one CEO, and a $50 million system that couldn't figure out they were the same person. This wasn't just a data problem- it was the catalyst that revealed how deeply the gap between traditional analytics and modern AI had become. At AIS Engage, we didn't just bridge this gap. We obliterated it.
Every enterprise claims to be "data-driven," but most are drowning in metrics that don't matter. Across industries, companies have deployed sophisticated chatbots and AI solutions that generate millions of customer interactions daily. Yet when it comes to measuring impact, they're still counting messages and tracking response times- analytics that barely scratch the surface of true business intelligence. Without a deeper understanding of customer behavior and sentiment, these organizations risk missing out on invaluable insights that could drive meaningful growth and innovation.
The disconnect is fundamental. Companies have invested heavily in AI capabilities, yet their measurement systems are outdated. It would be like owning a Ferrari but only tracking its fuel consumption- just as a high-performance vehicle requires advanced diagnostics to fully leverage its capabilities, businesses need sophisticated measurement systems to accurately assess and enhance their AI investments. The essential metrics that influence business decisions are often concealed beneath basic engagement data, resulting in missed opportunities for optimization and growth.
This analytics gap creates ripple effects throughout organizations. Marketing teams love to point to high interaction volumes while sales directors question lead quality. Customer service celebrates quick response times while product teams struggle to identify user pain points. Meanwhile, executives face a persistent challenge: proving the ROI of their AI investments. Traditional analytics dashboards, designed for web traffic and basic chat metrics, fail to connect AI conversations to actual business outcomes.
At AIS Engage, we recognized that the solution required more than just better data visualization. Companies needed a fundamental shift in how they approach AI analytics. Rather than adding another layer of metrics, we built a system that transforms raw conversation data into actionable business intelligence. By focusing on the context and nuances of conversations, we ensure that our insights are not only relevant but also deeply informed by the unique challenges each organization faces. Our approach bridges the gap between technical performance and business impact, delivering insights that drive real organizational change.
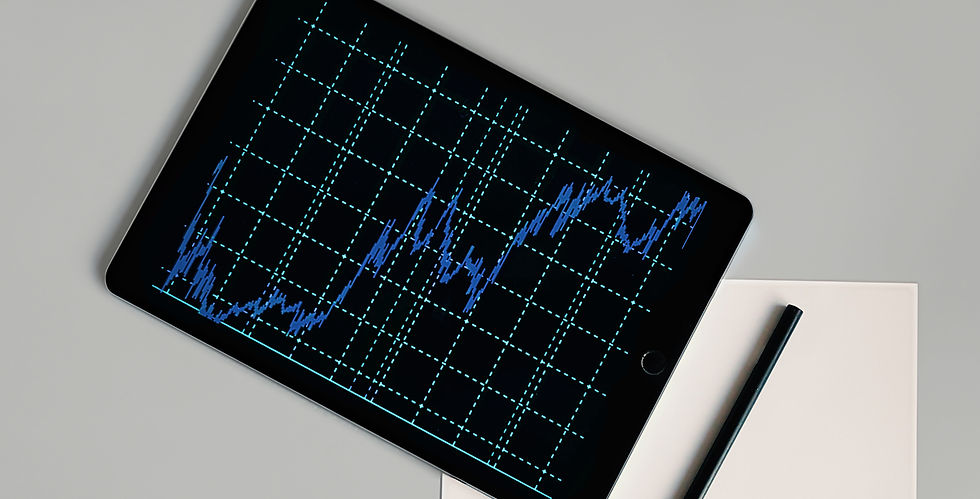
The Data Nightmare: When Excel Sheets Attack
Picture this: A Fortune 500 executive frantically clicking through five different spreadsheets at 2 AM, trying to figure out why his own name appears twelve different ways across his company's databases. Meanwhile, his newly hired customer service agent is telling customers that have been with them for over three years it can't find their orders because Jane Smith-Jones didn't match J.Smith didn't match JaneS93. This wasn't a hypothetical scenario- it was Tuesday at one of America's fastest-growing healthcare companies.
The roots of effective AI analytics run deeper than most realize. While other companies retrofit analytics onto existing chatbot solutions, our approach emerged from decades of data expertise. Our three AIS founders spent over 30 years in the trenches of enterprise analytics, witnessing firsthand how traditional measurement frameworks consistently failed to deliver actionable intelligence, which consistently stifled innovation and hindered organizations from making data-driven decisions.
First, there's the "collect now, analyze later" trap. Most businesses deploy AI solutions without considering how they'll measure success, leading to fragmented data collection and incomplete insights. This often results in missed opportunities for optimization and a failure to fully leverage the potential of AI technologies. At AIS, a radical decision was made to ensure that analytics would not be an afterthought; instead, it would drive the development process from day one. Every conversation flow, every user interaction point, and every data touchpoint is designed with measurement in mind.
The second challenge emerged from an unexpected source: data standardization. While companies invest heavily in AI capabilities, they often overlook the foundation of effective analytics - clean, consistent, accessible data. Our development process integrates sophisticated data normalization protocols directly into the conversation architecture. This means every customer interaction, whether it's a simple query or a complex multi-step process, generates standardized data that flows seamlessly into existing business intelligence systems.
But perhaps the most surprising obstacle we encountered wasn't technical at all - it was organizational. Companies struggle to maintain secure, centralized data repositories that both protect sensitive information and provide immediate access to authorized users. Our solution? We built a system where analytics isn't just a reporting tool - it's an interactive platform. Users can request performance metrics directly through the chat interface, while administrators access comprehensive dashboards that automatically organize data into relevant business contexts. This approach eliminates the traditional barriers between data collection and analysis, creating a continuous feedback loop that drives constant improvement.
Chaos Theory: How One CEO's "Multiple Personalities" Exposed a $2M Problem
Have you ever heard the well-known joke about the IT Director and his CEO? "Sir, according to our system, you don't exist," said the IT director to his CEO during a routine system check. "Well, technically you exist thirteen times, but none of them match your email signature." The CEO's response- unrepeatable in polite company- was very similar to the one that drove our wake-up call. When a company's own founder can't get past their database's convoluted data structure, something has gone fundamentally wrong with how we approach the data architecture. The solution would shape the way AIS Engage built the next generation of business analytics.
The unfortunately common "collect first, analyze when we have time" mindset nearly derailed a rapidly growing women's healthcare products company last year. Their initial request seemed straightforward: build an AI customer service assistant to handle order tracking and product recommendations. But during our discovery phase, we uncovered a data infrastructure that exemplified why analytics can't be an afterthought.
"What we found was a perfect storm of unstructured data," explains AIS Senior Solutions Architect. "The company had scaled from startup to mid-sized enterprise in just three years, but their data architecture hadn't kept pace. They were running their entire operation through a maze of Excel spreadsheets and SharePoint lists, held together by one overworked developer and some creative automation tools."
Without standardized data structures, even basic chatbot functions would fail. How could an AI assistant look up order status when order numbers appeared in different formats across multiple spreadsheets? How could it recommend products when product names weren't consistent? The chatbot would need to match customer information across systems, but with names entered a dozen different ways, simple tasks like order verification would become impossible. In one memorable instance, their CEO appeared as both "Robert Anderson" and "Anderson the Manderson" across different systems, causing payment reconciliation reports to constantly break. If their own CEO's name couldn't be standardized, how could we expect customer data to be reliable?
"Every attempt at building automated analytics had failed," continues our AIS Senior Solutions Architect. "Their dashboards would work for a week, then break when someone renamed a column or added free-text fields that didn't match existing patterns. Their lone developer spent more time fixing broken reports than improving their systems. The breaking point came during a test of their existing customer service workflows - we found that when customers asked about order status, their service team had to check up to five different spreadsheets, each with its own naming conventions and data formats. An AI chatbot would face the exact same roadblocks- garbage in, garbage out. Without standardized data, we'd just be building a faster way to get confused."
The Digital Bouncer: Teaching AI to Check IDs at the Door
Legacy data integration presented a unique challenge that demanded an unconventional solution. While other AI companies focus on post-processing data cleanup, AIS took a radically different approach. The industry standard of collecting data first and cleaning it later is fundamentally backward - by the time data hits your database, it's already too late: trying to standardize millions of data points after collection was like attempting to alphabetize a library after an earthquake. The real innovation lay in preventing the chaos before it began.
Enter our pre-validation architecture- a sophisticated system that earned an unexpected nickname from our development team: "The Digital Bouncer." You can think of it like a bouncer at an exclusive club: instead of letting everyone in and trying to sort out problems later, we check credentials at the door. Every piece of data- whether it's a customer name, date, or product code - goes through intelligent validation before it's allowed into the conversation flow.
The technical implementation combines a custom validation framework with seamless enterprise system integration. When a customer interacts with our chatbot, their inputs are instantly checked against multiple data sources through secure API connections. Names are standardized through natural language processing, dates are automatically formatted to match enterprise standards, and product codes are verified against live inventory systems - all in milliseconds. Our system can recognize "Bob A.", "Robert Anderson", and "Anderson the Manderson" as the same person, standardize the format, and maintain that consistency across every interaction, all without the user ever knowing it happened.
The security implications of this architecture are significant. By validating data at the entry point, we create an additional layer of protection against injection attacks and data manipulation. The system maintains detailed validation logs, creating an audit trail of every standardization decision while keeping sensitive information secure. More importantly, every validation decision feeds back into our system, making it smarter over time. When new variations of product codes or customer name formats appear, our system learns to recognize and standardize them automatically, building institutional knowledge with every interaction.
This approach has transformed how our clients handle data across their entire organization. The women's healthcare company saw their data consistency rates jump from 68% to 99.3% within three months. More importantly, they could finally trust their analytics dashboards, knowing that every piece of data was properly formatted and verified from the moment it entered their system.
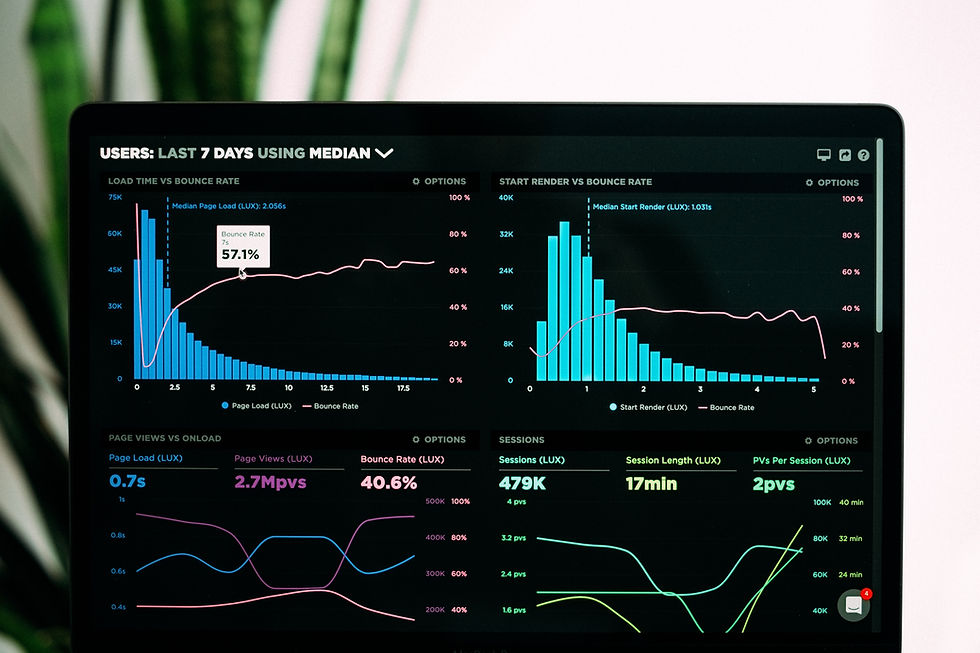
The Digital Bouncer: Teaching AI to Check IDs at the Door
While our pre-validation architecture solved the problem of new data entering the system, we took it upon ourselves to see if we could help with their problem of historical information. The women's healthcare company had three years of unstandardized data that their chatbot needed to access for real-time customer interactions. Without standardization, the chatbot could fail to match customers with their order history or understand product references in previous support tickets, and it could potentially require significant delays before their team could rectify the issues within their existing mock-database.
Typically, AIS Engage leverages advanced API integrations and custom HTML components to create an intelligent middleware layer that performs real-time data standardization. When a customer interacts with our chatbot and references historical information, the system performs just-in-time standardization through a series of secure connections. Rather than attempting to standardize entire databases at once, our chatbot creates standardized views of data on demand. We thought we might be able to apply this approach to their data dilemma.
Consider a common customer service scenario: A customer asks about their previous order of prenatal vitamins, but their name appears differently across multiple systems. Jane Smith-Jones might be logged as "J. Smith" in the order system, "Jane S Jones" in the CRM, and "Jane Smith-Jones" in support tickets. But with this company, our chatbot couldn't just search for exact matches- we found it would need to create temporary standardized views of customer data through secure API calls, matching standardized profiles against standardized order records in real-time. The customer gets accurate information instantly, while behind the scenes, the system is building connections between disparate data sources.
We realized that we'd need the chatbot to maintain these standardized connections in its conversation memory, building a growing network of validated data relationships. Once it learned the correct way to match a customer's various system identities, it automatically applied that knowledge to future interactions. This created an evolving bridge between legacy systems and modern customer interactions, without requiring massive database overhauls. It was such an effective approach that we have used it across every project since and made it an essential hallmark of the services AIS Engage offers.
Most importantly, this approach allows companies to maintain business continuity during the transition. AIS Engage can handle customer inquiries accurately while simultaneously improving data standardization through natural interactions. Each conversation adds to the system's understanding, leading to progressively better performance. Typically, within six months, over 85% of frequently accessed historical data is automatically standardized through normal customer service operations.
Small Beginnings to Big Steps: Expanding from Square One
Carrying the mix of our pre-validation architecture with the self-evolving database standardizer across all of our chatbot implementations brought us national recognition for our unique analytics approach. While companies traditionally rely on retrospective business intelligence platforms for their analytics, these tools share a common weakness: they analyze data long after customer interactions occur. The women's healthcare company was spending thousands of hours reconciling data across systems just to generate basic customer behavior reports. By the time insights reached decision-makers, the data was often weeks old and the standardization issues we discussed earlier made many reports unreliable.
Our chatbot architecture transforms this paradigm by turning every customer interaction into an immediate analytics opportunity. Through our secure API integrations and custom analytics components, AIS Engage doesn't just standardize data- it simultaneously captures, categorizes, and analyzes behavior patterns in real-time. The system's conversation flows include embedded analytics triggers that feed into our custom dashboard, giving stakeholders immediate visibility into customer interactions.
The difference becomes clear in practical application. When a customer mentions difficulty finding specific prenatal vitamin information, our chatbot doesn't just standardize their data and provide an answer- it immediately categorizes this as a navigation issue, maps it against similar complaints, and feeds this insight directly to our real-time dashboard. The standardization work we implemented means these insights are consistently formatted and immediately actionable, without requiring additional data cleaning or processing.
The impact on decision-making has been revolutionary. The healthcare company's marketing team used to wait weeks for reports to adjust their product placement strategy. Now they can see emerging trends as they happen through our custom analytics interface. When a new product launches, they can track customer reception and understanding in real-time, making adjustments to messaging and placement within hours instead of weeks.
The results speak for themselves. The company reduced their legacy analytics platform costs by 60% while achieving a 43% faster time-to-action on customer insights. More importantly, their ability to respond to customer needs in real-time led to a 28% increase in successful product recommendations and a 35% improvement in first-time purchase completion rates. The combination of clean, standardized data and instant analytics transformed what was originally intended as a customer service chatbot into a powerful business intelligence tool.
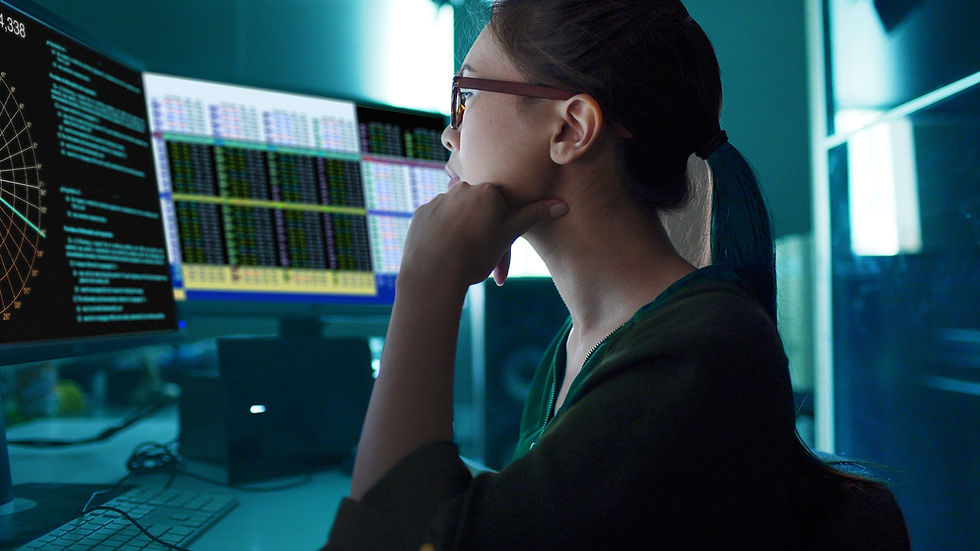
Reaching the Masses: How We Made Analytics "Trendy" Again
The healthcare technology sector isn't known for surprising success stories. Between regulatory compliance requirements and legacy system constraints, innovation typically moves at a glacial pace. So, when our implementation began generating headlines at major healthcare technology summits, even our team was caught off guard. The questions from industry leaders shifted from "How do you handle HIPAA compliance?" to "How did you achieve real-time analytics without disrupting existing workflows?" The unique combination of real-time data standardization and instant analytics capabilities demonstrated a new approach to healthcare technology that hadn't been seen before.
This recognition stemmed from our measurable results: the dramatic reduction in analytics costs coupled with improved data accuracy and speed-to-insight created a compelling narrative. The women's healthcare implementation became our flagship case study, demonstrating how conversational AI could bridge the gap between customer interaction and business intelligence while maintaining the highest security standards. By year-end, our innovative approach to healthcare analytics had attracted attention from multiple healthcare networks and pharmaceutical companies facing similar challenges with data standardization and real-time analytics. Our solution had identified and filled a crucial gap in the healthcare technology landscape.
A Data Standardization Miracle: What Happens When AI Gets Its Act Together
Enterprise digital transformation typically comes with a warning: prepare for disruption. Systems go offline. Databases freeze. Productivity grinds to a halt. But what if the most powerful transformations could occur without a single minute of downtime? Our healthcare client's story proves it's possible. While their systems continued operating normally, our AI architecture was quietly rebuilding their entire data ecosystem, one interaction at a time.
The transformation we achieved for the women's healthcare company represents more than just a successful implementation - it's a blueprint for how modern businesses can leverage conversational AI to solve complex data challenges. By combining real-time data standardization with immediate analytics capabilities, we've demonstrated that chatbots can do more than just answer questions - they can drive business transformation.
The metrics tell the story: 85% of historical data standardized through natural interactions, 60% reduction in analytics costs, 43% faster time-to-action on insights, and a 35% improvement in purchase completion rates. But perhaps the most important number is zero - the number of systems we had to take offline during implementation. Our architecture proved that major digital transformations don't have to disrupt business operations.
Looking ahead, we're already working on expanding these capabilities. The next generation of AIS Engage will include enhanced predictive analytics features and deeper integration capabilities, all while maintaining our commitment to security and real-time performance. The success of this implementation has shown that the future of business intelligence isn't in static reports or delayed analytics - it's in the immediate, interactive insights that only conversational AI can provide. As we continue to evolve our platform, we remain focused on our core mission: turning every customer interaction into an opportunity for business improvement.
Comments